SCAF: A Speculation-Aware Collaborative Dependence Analysis Framework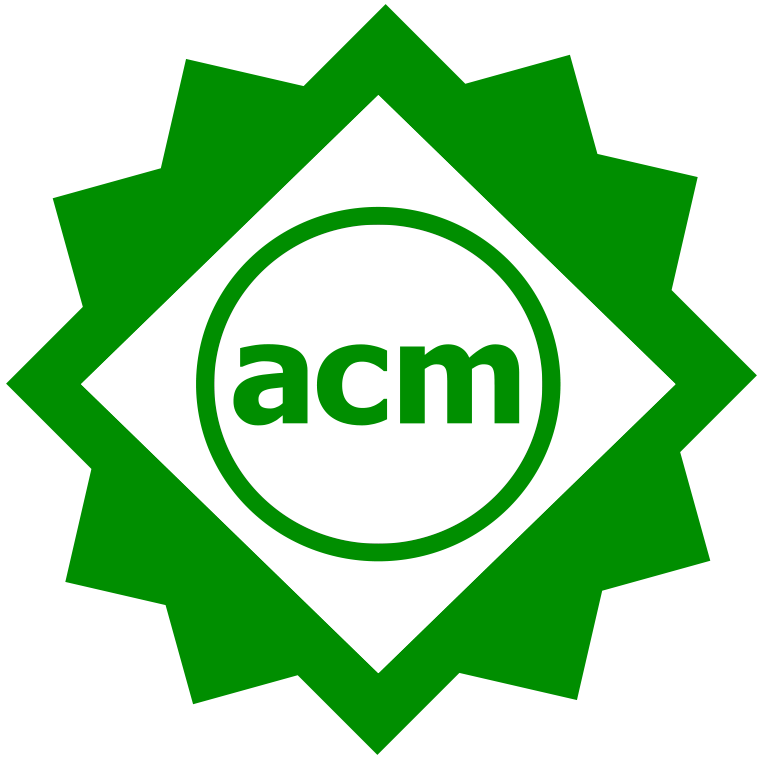
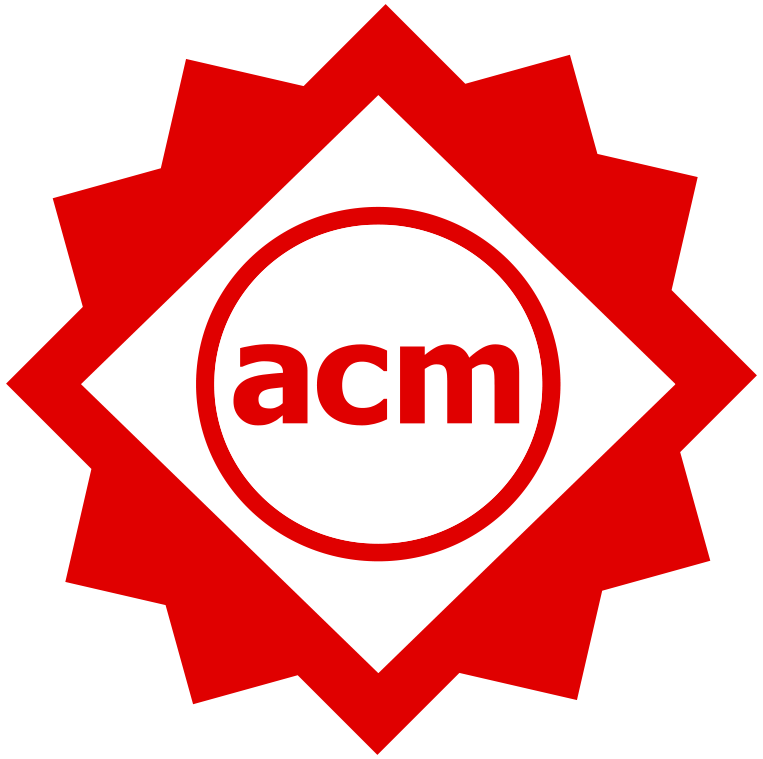
[abstract] (ACM DL, PDF)
Sotiris Apostolakis, Ziyang Xu, Zujun Tan, Greg Chan, Simone Campanoni, and David I. August
Proceedings of the 41st ACM SIGPLAN Conference on Programming Language Design and Implementation (PLDI), June 2020.
Awarded all top ACM Reproducibility Badges offered by the Artifact Evaluation Committee.
Program analysis determines the potential dataflow and control flow
relationships among instructions so that compiler optimizations can
respect these relationships to transform code correctly. Since many
of these relationships rarely or never occur, speculative
optimizations assert they do not exist while optimizing the code. To
preserve correctness, speculative optimizations add validation checks
to activate recovery code when these assertions prove untrue. This
approach results in many missed opportunities because program analysis
and thus other optimizations remain unaware of the full impact of
these dynamically-enforced speculative assertions. To address this
problem, this paper presents SCAF, a Speculation-aware Collaborative
dependence Analysis Framework. SCAF learns of available speculative
assertions via profiling, computes their full impact on memory
dependence analysis, and makes this resulting information available
for all code optimizations. SCAF is modular (adding new analysis
modules is easy) and collaborative (modules cooperate to produce a
result more precise than the confluence of all individual results).
Relative to the best prior speculation-aware dependence analysis
technique, by computing the full impact of speculation on memory
dependence analysis, SCAF dramatically reduces the need for
expensive-to-validate memory speculation in the hot loops of all 16
evaluated C/C++ SPEC benchmarks.